If you try to stay informed about things, you'll undoubtedly run across an increasingly desperate experience with election polls.
Everywhere you look, pollsters show a near 50/50 split between the two major political parties, only ever varying by the margins of error that are part and parcel of statistical sampling.
It's a desperate experience because pollsters need attention to generate revenue, even though revenue is not directly tied to relevance insofar as whether polls are relevant to our understanding of how our neighbors might behave.
That's why my recommendation to you is this: ignore election polls, because the only polls that matter are exit polls (the ones taken after someone literally just got done voting).
In this article, I go into detail about the four reasons you should ignore election polling:
- Poll data doesn't actually tell us how people will vote
- Tight races are better for advertising revenue
- Polls don't account for what can't be measured
- Poll gaps are much wider than what's being reported
There's also an Election Polling Simulator you can use, with plenty of instructions and examples to play around with—but I highly suggest you read the article first.
Alright, let's get started:
Poll data doesn't actually tell us how people will vote
Pollsters don't want to underestimate or overestimate candidates like they have done before. When pollsters make strong predictions and back up those predictions with statistical artifacts, they create a one-way, fragile relationship of consumer trust. If the pollsters end up being right, the relationship is unchanged. If, however, the pollsters are wrong, the fragility of this relationship is exposed and consumer trust in the pollster is lost.
It's a difficult situation for pollsters to be in. Do they fine-tune their statistical models and make educated predictions about a future in which they could be wrong? Or do they adjust their statistical tools so that they produce inferential data that is palpably pleasant to all sides of American politics?
Think about it: if I tell you that, according to my calculations, Candidate A has a 99.99% chance of winning, what would happen to your faith in my prediction capacity if Candidate A ends up losing? You'd likely lose some, if not all, faith in my ability to predict an election outcome.
But is that true for every prediction I make?
Let's say I adjust my models for a new election, and now I say that Candidate A has a 62.5% chance of winning. How does this new number affect your understanding of my mathematical model of future human behavior? It's a higher chance than a coin flip, sure, but not so egregiously high that you might become suspicious. In fact, you might even feel a bit of relief—after all, greater than 62.5% feels more believable than 99.99%, doesn't it?
As it turns out, we are predisposed to believing moderate predictions more than non-moderate predictions because of a phenomenon known as The Illusion of Validity:
A cognitive bias in which we overestimate the accuracy of our predictions when analyzing data, especially when the data seems to suggest a particular outcome.
The illusion of validity creates a false sense of confidence in someone's ability to interpret and predict outcomes when they only have a moderate amount of information.
In the context of election polls, a prediction of 62.5% feels more valid than 99.99% because it aligns with our intuitive understanding of the complexities and uncertainties involved in predicting human behavior. We subconsciously recognize that elections are influenced by numerous factors, so a more modest prediction feels more considerate of these complexities.
So what happens when we update our models again, and now our prediction for Candidate A hovers around 50%?
Suddenly, the momentary relief from a modest yet favorable estimate vanishes, and we find ourselves in a conundrum:
- A more mathematically neutral estimate of the election outcome, where each candidate has about a 50% chance of winning, yields the same statistical probability of winning for each candidate, and yet...
- ... seeing that our candidate has "only" a 50% chance of winning creates a heightened state of anxiety in our minds.
In other words, we sometimes feel like 50/50 odds are less favorable to our candidate, despite both candidates having the same likelihood of winning. Interestingly, the same parts of our brains that helped our ancestors survive in the wilderness is the same part of our brains that is causing us to underestimate the likelihood of a favorable outcome. When a shadow flickers by, was it a branch from a tree in the moonlight? Or was it a deadly predator? Our brains are hardwired to assume the worst in order to maximize survival likelihood.
But we aren't trying to survive in the wilderness, here—we're trying to feel some reassurance about the upcoming election. So what do we do?
The answer, fortunately or unfortunately, is to ignore all the polls and anyone reporting polls until after the election. I'm not telling you to do this because of internal cognitive biases that we cannot control (although that's one reason to consider swiping past any election polling content until November), but rather, because when attention = engagement
and engagement = revenue
, we have to assume that all reporting is at least somewhat motivated by maximizing attention engagement.
Tight races are better for advertising revenue
Consider the impact of consistently reporting election races as hovering around 50/50 odds:
- What kind of emotional state are viewers going to be in?
- Are people going to feel more informed about the potential outcomes of an election if polls report 50/50 odds?
- Are people going to be more engaged with the media content that reports such a harrowing, close race?
Media outlets reporting tight elections are maximizing attention engagement by keeping viewers in the Area of Maximum Concern:
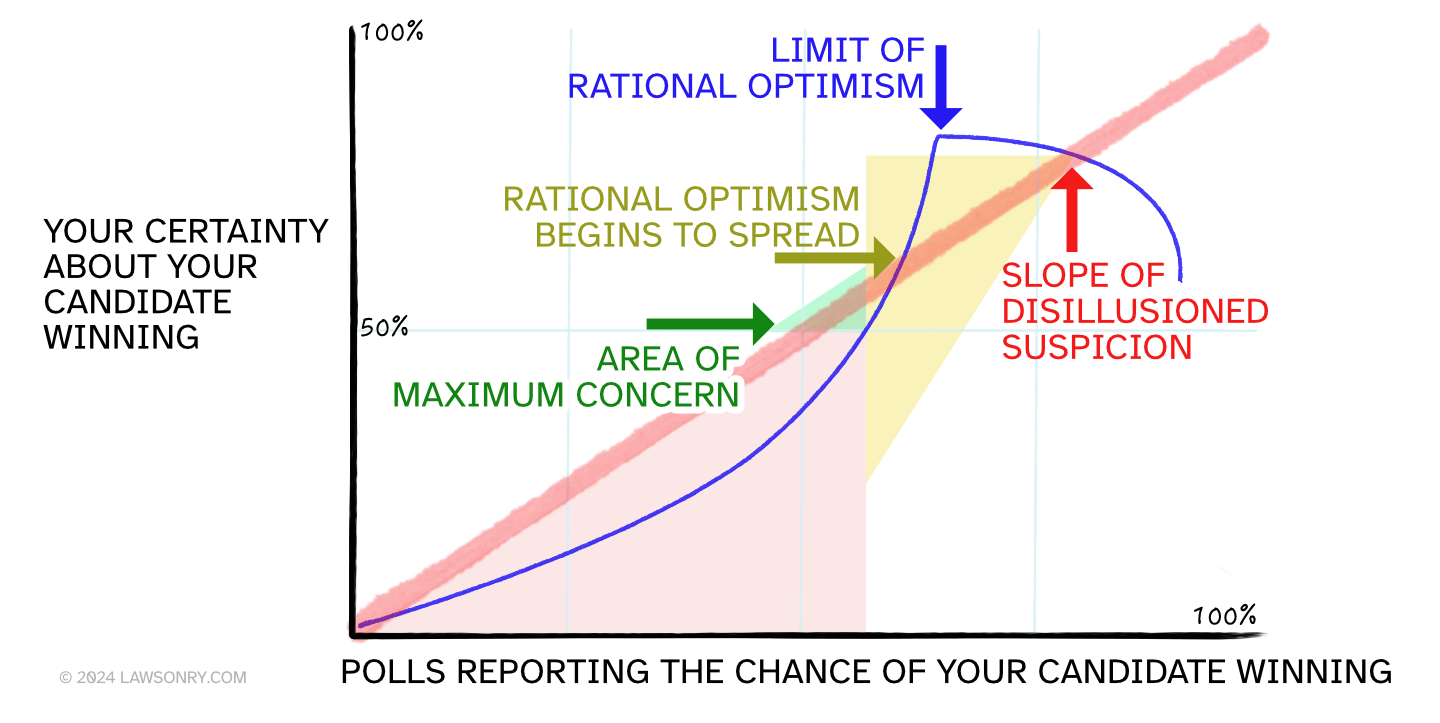
Keeping people in a state of maximum concern enables suggestibility, hyper-partisanship, and performative in-group behavior. This is good for engagement—but bad for social reality construction. That's because polls do not predict the future; polls model mathematical uncertainty of human behavior based on past data.
How would ratings be affected—how many less clicks would media outlets get—if they reported polls that were boring?
On social media, revenue is maximized when attention engagement is maximized, and attention engagement is maximized when people have a heightened sense of anxiety about what they are engaging with. On top of that, the context of an election, not everyone has the goal of an informed electorate. There are just too many competing and overlapping forms of attention manipulation at play here to confidently say whether a media outlet's reporting of election polls represent reality—or if they're more focused on monetizing viewership at the expense of your anxiety.
In this kind of decision-making environment, there's no new information that is going to be gained by following election polls for the next two weeks.
Polls don't account for what can't be measured
Polls use "Likely Voter" (LV) models to determine who to contact. LV models, like all statistical data, are based on past data; statisticians look at who participated in previous elections and then make educated guesses as to who might participate in an upcoming election. there are no guaranteed ways to measure actual people who will be voting, nor can polls measure intensity or drive.
New voter registration is helpful in determining where significant anomalies may exist–anomalies that can help provide the kind of context to election polls that we need in order to understand them, but that are often left out by media organizations are are not reporting polls for the purposes of clarity.
Of course, there are caveats to using new voter registration as any indication of anomalous electorate behavior, most notably because some states incorporate voter registration with other forms of government services. In California, for example, you can register to vote while renewing your driver's license.
Where new voter registration does provide insights is when there are correlational variables that may end up being causal. For example, after the Supreme Court overturned Roe v. Wade, new voter registration surged in many states. Turnout in response to things like this are not something that we can directly measure until after the election—which means that polling data can only guess how votes will be cast, but they cannot predict who will win the election.
Some polls even use Registered Voter (RV) models, but not all registered voters vote in an election. This creates a multi-layer statistical inference issue where one must account for two things:
- the likelihood of the sampled population being an adequate representation of the total population, and
- the likelihood of the sampled population to actually get out and vote.
This is important to consider, seeing as how poll reporting tends to include both kinds of sampling methods. Take, for example, the below snapshot from FiveThirtyEight's presidential election polls in Pennsylvania, a key battleground state for any candidate:
Example polls
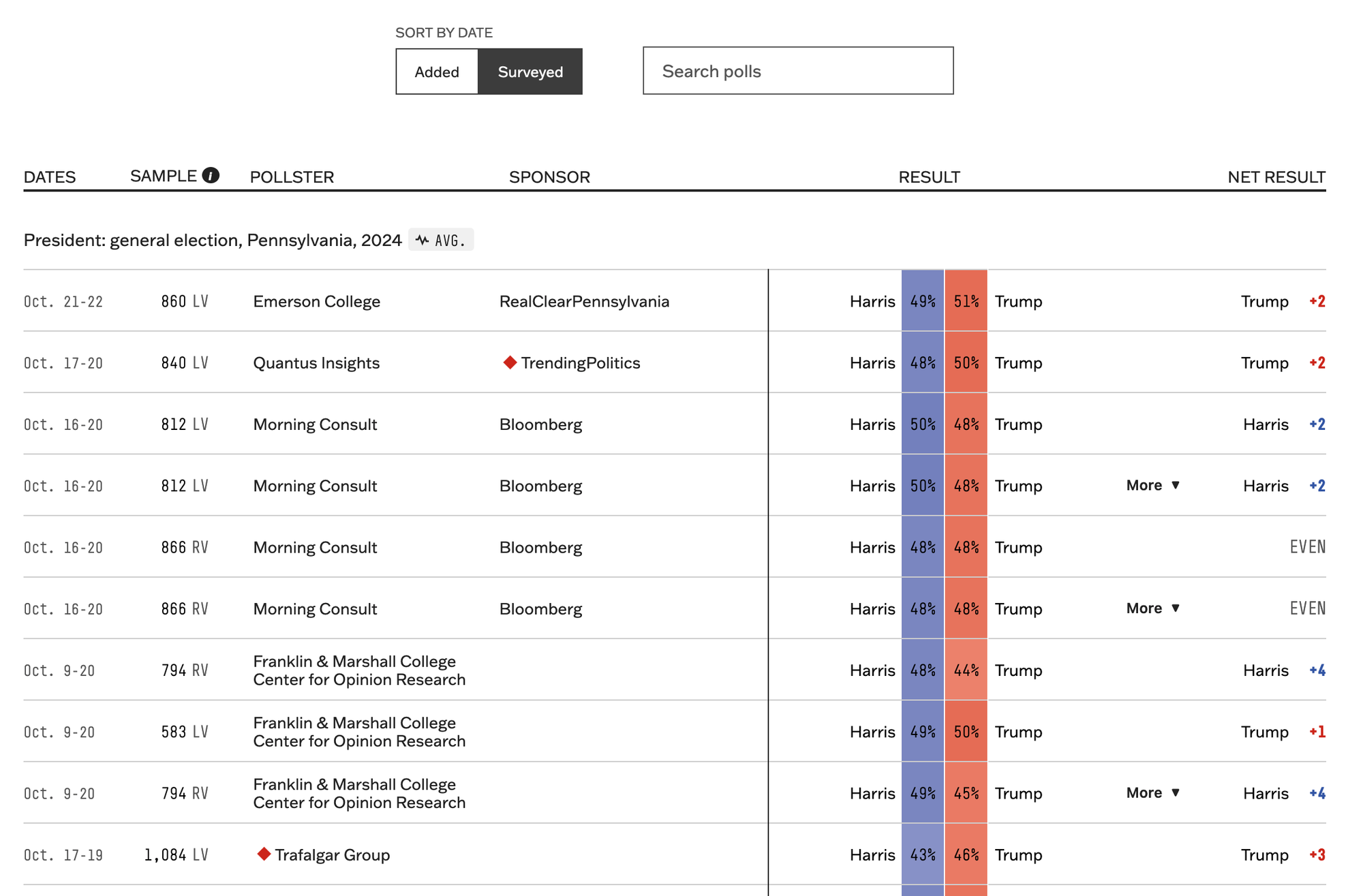
Of the ten polls listed, four of them use Registered Voter models. Keep that in mind for when you're using the election simulator, as "are you registered to vote" is a different frame of reference from "how do I know you're going to vote" (as well as the best way of understanding voter behavior: "how did you vote" (past tense, since we'll only have new information once the election is over)).
Poll gaps are much wider than what's being reported
Polls often report their data with a 95% confidence interval.
Think of a 95% confidence interval like this: if we went out and took 100 different samples of voters and asked each group the same questions, we expect about 95 of those samples to give us results that match what's really happening in the whole population. But here's the thing: when we only do one poll, we can't know for sure if we got one of those 95 good samples or one of the 5 bad samples. (They're bad samples because they don't accurately reflect the total population).
It's kind of like trying to guess what's in a huge jar of different-flavored jellybeans by only looking at a handful. If you took 100 different handfuls, about 95 of them would give you a pretty good idea of the distribution of flavors in the whole jar. But when you only get to look at one single handful, you can't be certain whether you're seeing a mix that really represents what's in the whole jar or a biased sampling of the whole population.
Professor Don Moore, who runs the Accuracy Lab at UC Berkeley, analyzed 1,400 polls across 11 election cycles and found that confidence intervals in election polling was way off track. He and one of his research assistants shared the following in an interview:
Most polls report a 95% confidence interval. But we found that the actual election outcome only lands inside that interval 60% of the time—and that’s just a week before the election. Further out, the hit rates fall even farther. Polls taken a year before the election stand only a 40% chance of getting the vote share from the actual election inside the poll’s 95% confidence interval.
Let’s say a candidate is polling at 54% a week before the election, with a margin of error of plus-or-minus 3%.
The 95% confidence interval implies a 95% chance that the candidate will win 51% to 57% of the vote.
Our analysis shows that in reality, you’d have to double that margin of error to plus or minus 6% to get 95% accuracy.
That means that the outcome is less certain; the candidate is likely to get anywhere from 48% to 60% of the vote.
I've created a calculator you can use that helps to illustrate this:
Realistic Poll Margins Adjustment Calculator
Enter in any reported Harris or Trump percent from poll reporting (for example, you could use the ten polls reported in the Example Polls from earlier). Notice how the actual range of the poll is much wider when we use a more appropriate margin of error based on Moore's research.
This calculator above is one example, but there are a lot of other variables—sampling bias and true population split—that factor in as well. Which brings me to...
The Election Polling Simulator
Get StartedGo ahead and tap or click the "Get Started" button to open up the Election Polling Simulator. At the top you'll see instructions. Scroll down when you get there and you'll see some guidance for exploration.
Really? Just "ignore the polls"?
Yes.
Media outlets reporting on election polls that converge around a coin flip's odds are, whether they know it or not, maximizing audience engagement by exploiting how our brains respond to stress.
When faced with maximum uncertainty (like a true 50/50 odds split), we experience a compelling urge to seek more information—to resolve the uncertainty that makes us so uncomfortable.
Media companies and social media platforms, operating within the attention economy, are well aware of this psychological tendency. By maintaining a narrative of neck-and-neck races, they effectively keep us in a constant state of maximum uncertainty.
This isn't just engaging–it's addictive.
The human brain, wired to resolve uncertainties for survival, keeps coming back for more information, more updates, more analysis—and each click, each minute spent watching, each page load on their websites translates directly into revenue. It's a strategy that exploits our psychological need for certainty and transforms it into a steady stream of engagement and profit.
Sometimes statistics feel like they should give us a sense of relief. After all, they're mathematical models built with logic and rules—surely there's something we can gain from them! Unfortunately, given the context in which election polls are reported to us, their effect is actually the opposite of relief.
So: Really. Yes. Please. Just ignore the polls.
Are you on BlueSky? Share your thoughts about this article with me: jesselawson.bsky.social